TL;DR: Semi-autonomous AI agents represent the next evolution in legal operations technology, moving beyond basic automation to handle complex multi-step workflows. Experts from Cedar, UpLevel Ops, Magic Leap, and Ruli AI outline implementation strategies for AI agent deployment, including knowledge management fundamentals, governance frameworks, oversight mechanisms, and the balance between horizontal enterprise tools and vertical legal-specific solutions. The panel addresses critical concerns about data training, security, and the strategic role of legal operations professionals in championing AI adoption.
As legal departments face increasing demands with limited resources, AI agents offer a promising solution for scaling support and streamlining operations. At our recent SDSF event, a panel of legal technology experts shared practical insights on implementing AI agents in legal operations—from governance considerations to specific use cases and future possibilities.
The legal profession is at a pivotal moment in its technological evolution. While generative AI tools have gained widespread adoption for content creation and research, the next frontier appears to be AI agents—semi-autonomous digital workers that can handle multi-step workflows with minimal human intervention. For legal operations teams looking to scale their support capabilities, these agents represent both an opportunity and a challenge.
Meet our expert panel
Our discussion featured insights from leaders representing various perspectives within the legal technology ecosystem:
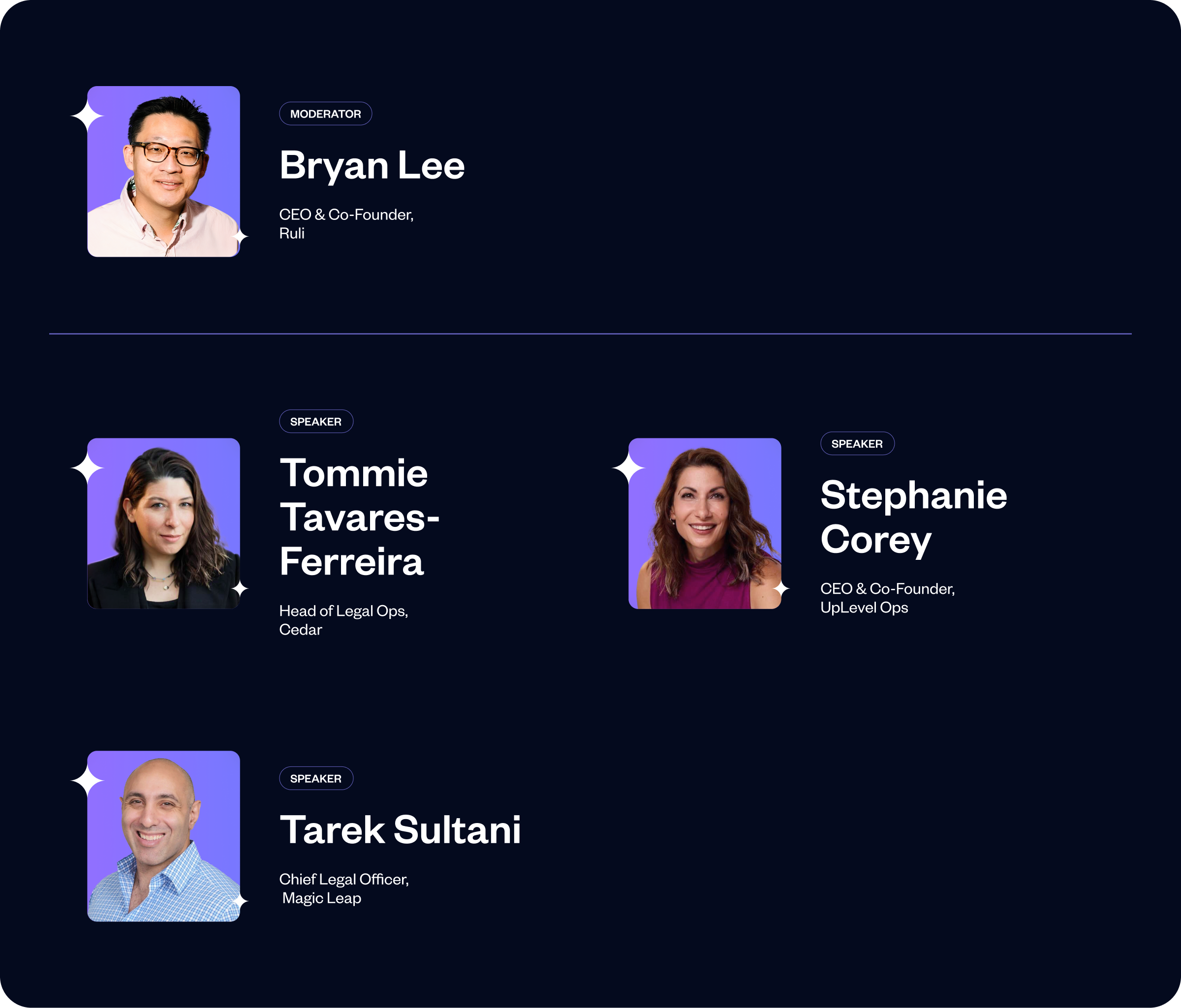
- Brian Lee (Moderator), Co-founder and CIO of Ruli AI
- Tommie Tavares Ferreira, Head of Legal Operations at Cedar
- Stephanie Corey, Co-founder and CEO of UpLevel Ops
- Tarek Sultani, Chief Legal Officer at Magic Leap
AI readiness: Preparing your department for agent implementation
Before implementing AI agents, organizations need to assess their readiness and establish appropriate governance frameworks. Our panelists emphasized that while some aspects of AI readiness mirror traditional technology implementations, AI agents present unique considerations.
Action plan: Laying the groundwork for AI agents
1. Start with knowledge management fundamentals
- Audit and organize your existing knowledge base
- Document processes that could be automated
- Identify where your information currently resides across systems
- Standardize templates and playbooks
As Tommy Tavares Ferreira noted: "If you actually want the responses to be good, you need good data in, good data out."
2. Evaluate your current workload
- Conduct a value exercise to identify repetitive, low-value tasks
- Use the Eisenhower matrix (urgent vs. important) to prioritize potential use cases
- Consider whether work should be done at all before automating it
Steph Corey advised: "We all hear 'do more with less.' You know, it's exhausting to continuously hear that. How about doing less? Like, do we need to be doing this work?"
3. Develop a staged implementation approach
- Start with small, well-defined use cases
- Implement in phases rather than attempting a comprehensive solution
- Focus on quick wins that demonstrate value
Corey emphasized: "Baby steps, baby steps, baby steps. When you look at the work coming to the department, one exercise that we do is we call it a value exercise... that will really help bubble up opportunities."
4. Establish AI governance frameworks
- Define responsible use policies for AI tools
- Determine appropriate oversight mechanisms
- Create security and privacy guidelines
Tarek Sultani shared: "When I think about how we craft a policy and how we think about responsible use for our enterprise... The tool can do everything right, but if people are not using it responsibly, if the employees are not using it responsibly, you're gonna still have a problem."
Understanding AI agents: Beyond chatbots
AI agents represent the evolution from simple chatbots to more sophisticated digital workers that can manage end-to-end processes with minimal human intervention.
Key characteristics of AI agents in legal operations:
1. Semi-autonomous operation
- Capable of handling multi-step workflows
- Can make certain decisions within defined parameters
- Knows when to escalate to human oversight
2. Integration with existing systems
- Connects with knowledge bases, document repositories, and other tools
- Creates a unified experience across fragmented systems
- Provides a conversational interface to complex backends
3. Continuous improvement capabilities
- Learns from interactions to improve responses
- Can be trained on organization-specific data
- Adapts to changing requirements
Practical use cases: Where AI agents deliver value today
The panel explored several practical applications where AI agents can provide immediate value to legal operations teams.
Action plan: Implementing high-impact AI agent use cases
1. Front-door legal intake and triage
- Create agents that serve as the first point of contact for legal requests
- Automatically route matters to appropriate attorneys based on subject matter
- Answer common questions without human intervention
- Capture essential information before escalating complex matters
2. Knowledge management and self-service
- Deploy agents that make policies and procedures searchable
- Enable employees to get answers directly from corporate guidelines
- Reduce repetitive questions to the legal team
3. Document review and standardization
- Implement agents to review and clean up inconsistent policies
- Identify contradictions in existing documentation
- Suggest improvements to standardize language
4. Continuous process improvement
- Deploy agents to identify bottlenecks in legal workflows
- Gather metrics on common issues and request types
- Provide insights for operational improvements
The human-AI partnership: Oversight vs. autonomy
A significant discussion centered around finding the right balance between human oversight and agent autonomy—particularly regarding quality control and risk management.
Action plan: Balancing control and efficiency
1. Establish appropriate oversight mechanisms
- Determine which agent activities require human review
- Create sampling protocols for quality assurance
- Implement escalation paths for complex or high-risk matters
2. Apply risk-based oversight
- Implement stricter controls for high-risk domains
- Allow greater autonomy for low-risk, routine matters
- Adjust oversight based on demonstrated performance
3. Develop error recovery processes
- Create procedures for identifying and correcting agent errors
- Establish feedback loops to improve agent performance
- Manage stakeholder expectations regarding potential mistakes
4. Implement continuous training
- Use errors as learning opportunities to improve agent performance
- Create mechanisms to provide feedback to your AI systems
- Regularly update knowledge bases to maintain accuracy
Tarek Sultani emphasized: "Front and center of every policy I've had has been that human verification is a must. Nothing goes out the door without a human overseeing it. And if it's going to a client, we have two layers of human review."
The learning dilemma: Training on your own data
One of the most significant tensions discussed by the panel involved the balance between data security concerns and the need for AI systems to learn from an organization's data to become more effective.
Action plan: Resolving the training paradox
1. Understand different types of learning
- Distinguish between foundational model training and instance-specific learning
- Clarify how your AI tools use your data for improvement
- Identify which approaches align with your security requirements
2. Develop clear data usage policies
- Create guidelines for what data can be used for agent training
- Establish security controls around sensitive information
- Implement technical safeguards to prevent data leakage
3. Communicate benefits of personalized learning
- Help stakeholders understand how learning improves agent performance
- Demonstrate the value of organization-specific knowledge
- Provide examples of how learning prevents errors
4. Create controlled learning environments
- Implement sandboxed environments for AI training
- Use synthetic or anonymized data where appropriate
- Ensure learning happens within secure boundaries
Horizontal vs. vertical solutions: Enterprise-wide vs. legal-specific AI
As companies implement AI solutions, legal operations professionals must navigate the tension between enterprise-wide "horizontal" AI tools (like Microsoft Copilot or Google Gemini) and specialized "vertical" solutions designed specifically for legal use cases.
Action plan: Navigating the tool selection process
1. Assess enterprise vs. specialized needs
- Identify where general-purpose AI tools are sufficient
- Determine which functions require legal-specific capabilities
- Consider integration requirements with existing systems
2. Leverage existing enterprise investments
- Look for creative ways to adapt enterprise tools for legal needs
- Identify opportunities to use tools that are already approved
- Partner with IT to customize enterprise solutions
3. Make strategic tool selection decisions
- Choose tools that attorneys will actually use
- Focus on solving legal-specific problems first
- Consider adoption likelihood in tool selection
4. Navigate budget constraints creatively
- Look for AI budget allocations that can be directed to legal tools
- Consider phased approaches that start with enterprise tools
- Build business cases based on efficiency and risk reduction
The future of legal operations with AI agents
Looking ahead, our panelists shared optimistic visions for how AI agents will transform legal operations roles and create new opportunities for legal professionals.
Action plan: Positioning for the AI-enabled future
1. Develop AI expertise within legal operations
- Build skills in prompt engineering and AI management
- Become the AI champion within the legal department
- Position legal operations as the bridge between lawyers and AI
2. Anticipate evolving AI capabilities
- Monitor developments in agent capabilities and security
- Prepare for increasing autonomy in legal workflows
- Develop frameworks for managing more sophisticated agents
3. Focus on high-value human contributions
- Identify areas where human judgment remains essential
- Shift legal professionals toward strategic advisory work
- Create career paths that emphasize AI partnership
4. Prepare for organizational change
- Develop change management strategies for AI adoption
- Help attorneys understand how to work effectively with agents
- Create training programs that build AI competency
Steph Corey shared: "I get asked all the time for career advice from newer legal ops professionals and that is what I tell them. You can really cement yourselves in your role through AI. Most CLOs and GCs aren't sitting around playing with this technology. So the legal ops professional can really do this."
The path forward: Starting your AI agent journey
Implementing AI agents in legal operations requires careful planning, but doesn't need to be overwhelming. Our panelists emphasized taking incremental steps toward more sophisticated automation.
Action plan: Getting started with AI agents
1. Begin with a readiness assessment
- Evaluate your knowledge management maturity
- Identify potential use cases based on repetitive work
- Assess security and governance requirements
2. Start with discrete, well-defined pilots
- Choose a specific function for your first agent implementation
- Set clear success metrics for the pilot
- Gather feedback from users and stakeholders
3. Develop an AI roadmap
- Create a phased implementation plan
- Prioritize use cases based on value and complexity
- Align with broader organizational AI initiatives
4. Build internal AI expertise
- Identify team members who can champion AI adoption
- Invest in training for legal operations professionals
- Create partnerships with IT and data science teams
Conclusion: Embracing the AI agent revolution
AI agents represent the next evolution in legal operations technology—moving beyond basic automation to create intelligent digital workers that can handle complex, multi-step workflows with minimal human intervention. While concerns about oversight, data security, and integration remain valid, the panelists emphasized that these challenges can be overcome with thoughtful planning and implementation.
As Steph Corey aptly summarized, the key is to take "baby steps" toward implementation, focusing on well-defined use cases that deliver immediate value while building toward a more comprehensive AI strategy. By positioning legal operations professionals as AI champions within their organizations, teams can harness the power of these technologies to transform legal service delivery.
The future of legal operations likely belongs to those who can effectively partner with AI agents—combining human judgment and creativity with AI efficiency and consistency to deliver better legal services at scale.
This blog post is based on the panel discussion "Leveraging AI Agents for Scalable Support in Legal Operations" from our recent SDSF event, featuring Tommy Tavares Ferreira (Cedar), Steph Corey (UpLevel Ops), and Tarek Sultani (Magic Leap), moderated by Brian Lee (Ruli AI).